Building AI Agents for Enterprise vs. Consumer
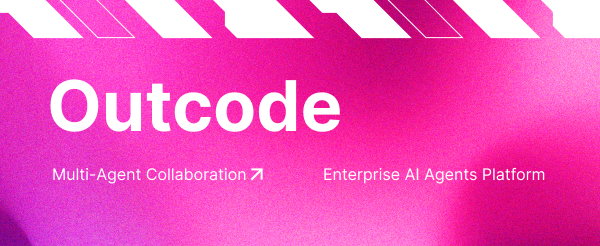
โ
In 2025, AI agents are evolving from everyday assistants to the core infrastructure of enterprise operations. We are doing more and more of our work with AI, and the need for enterprise-grade AI agents that are autonomous, self-aware, proactive, and connected to real-world operational systems is rapidly growing beyond reactive tools that simply respond to user requests.
But there's an important question here:
"CAN A GENERIC AI AGENT BE USED FOR ENTERPRISE TASKS?" AND "CAN EVERYDAY AI TOOLS HANDLE SOPHISTICATED TASKS IN THE ENTERPRISE?"
The correct answer is no.
Enterprise environments have a higher level of complexity, unique requirements, and clearer lines of accountability than our personal lives. In this article, we'll discuss these differences, why typical AI agents have limitations in the enterprise, and why an Enterprise Agentic Platform is essential.
1. the nature of the purpose of use is different
TYPICAL AI AGENTS ARE DESIGNED PRIMARILY TO IMPROVE PERSONAL CONVENIENCE AND PRODUCTIVITY. THEY PERFORM RELATIVELY SIMPLE, TASK-ORIENTED FUNCTIONS LIKE MANAGING SCHEDULES, SUMMARIZING CONTENT, OR ANSWERING SIMPLE QUESTIONS.
ENTERPRISE AI AGENTS, ON THE OTHER HAND, HAVE AN ENTIRELY DIFFERENT SET OF NEEDS. THEY NEED TO UNDERSTAND AND EXECUTE COMPLEX WORKFLOWS TO HELP ORGANIZATIONS RUN MORE EFFICIENTLY AND IMPROVE PERFORMANCE.
For example, not just invoice processing:
- Production planning based on demand forecasting
- Supply chain realignment tied to real-time sales data
- Run conditional automated approval processes based on budget control criteria
- MANAGE THE MOVEMENT OF DOCUMENTS AND DATA BETWEEN MULTIPLE SYSTEMS, INCLUDING INTERNAL SYSTEMS, SAP, EMAIL, ETC.
- Human-in-the-loop collaboration, where humans see the results of AI's work and key data in real time and can co-direct judgment or proactively intervene in exceptional situations, to maintain decision quality while continuing automation.
To be truly enterprise-ready, AI agents must be able to autonomously handle sophisticated operational tasks that involve autonomous decision-making + system integration + exception handling + human intervention. Therefore, enterprises don't need AI as a mere assistant, but rather autonomous agents as "operational entities" that organically execute
business processes, and an Enterprise Agentic Platform to support them.
2. single task vs. complex workflow
Typical AI is simple, task-oriented, and driven by direct commands from users. Enterprise applications, on the other hand, need to be able to understand and manage complex flows. They need an "Agentic Workflow Layer" that can design and operate these complex structures, which is what the Enterprise Agentic Platform is all about.
Off-the-shelf chatbots vs. organization-specific orchestration
General-purpose chatbots are sufficient for consumer AI, but businesses need enterprise-level orchestration to meet a variety of needs:
- CONNECT WITH APIS, CRM, ERP, DW, EMAIL, SLACK, AND MORE
- Process status tracking and automated reporting
- Fine-tune your data to understand your organization's context
At the end of the day, it's not about "talk," it's about "action.
3. Security, compliance, and accountability
Consumers only need a relatively simple privacy level of security:
In contrast, enterprise environments require the following elements
- Data Isolation: Customer-specific, department-specific, and project-specific data should be completely isolated, and the
- FINE-GRAINED ACCESS CONTROL AND ROLE-BASED AUTHORIZATION (RBAC): YOU NEED TO HAVE FINE-GRAINED CONTROL OVER WHO CAN SEE WHAT INFORMATION AND RUN WHICH AGENTS
- Audit Trail: A historical record of when an agent made a decision, what decision it made, and what data it acted on.
- Explainability: Ensure explainability and transparency of AI's decisions and behaviors
- And most importantly, in an enterprise environment, you don't have a single agent, you have hundreds or tens of thousands of agents working in parallel.
- Without a management scheme that can centrally monitor, control, deploy, and audit all of these agents, agent-based operations are unsustainable in the real world.
To meet these demands, AI agents need to be structurally trusted and manageable, not just technically smart. The Enterprise Agentic Platform is not just a "tool," it's the infrastructure for an AI-driven operating system.
4. EXECUTION WITHOUT ANALYSIS IS DANGEROUS AI AGENTS WITH BUILT-IN PREDICTIONS AND JUDGMENT
Execution in the enterprise is not just repetition.
Most core tasks involve making decisions based on predictions, judgment, and analysis.
For example, in a demand forecast-based order automation scenario, you might use the
- AI COLLECTS SALES DATA AND
- ANALYZE TRENDS BY SKU
- Forecasting future demand
- Compare inventory levels to determine if an order is needed
- AUTOMATIC ENROLLMENT AND CONTACT NOTIFICATIONS IN ERP
WHAT'S IMPORTANT IN THIS FLOW IS THAT THE AGENT HAS BUILT-IN ANALYTICS AND JUDGMENT, SOMETHING YOU CAN'T ACHIEVE WITH SIMPLE CHATBOT-LEVEL AI.
Outcode provides an Agentic Workflow structure that allows AI to naturally perform "analyze โ decide โ act" within a workflow.
5. FOR AI TO REALLY "WORK," IT NEEDS TO BE CONNECTED: THE ROLE OF INTEGRATION
NO MATTER HOW GOOD AI IS AT MAKING DECISIONS, IT STILL NEEDS TO BE CONNECTED TO REAL-WORLD BUSINESS SYSTEMS TO EXECUTE.
- DOZENS OF CONNECTIONS TO ERP, EMAIL, SLACK, INBOX, CLOUD STORAGE, AND MORE
- MAKE API CALLS, TRIGGER RPA, UPLOAD FILES, SEND MESSAGES, AND MORE
- Security and permission management should also be built in
The middle infrastructure that connects these different systems is the Integration Platform.
๐ Enterprise Agentic Platform = AI + Orchestration + Integration
Without "Integration" of these, agents are disconnected from business systems and are powerless.
Outcode has a modular iPaaS structure under the hood, allowing agents to "do" real work, not just interactive responses.
** Outcode supports external LLM integration protocols, such as Anthropic's Model Context Protocol (MCP). However, Outcode's MCP is not the same as Multi-Agent Collaboration Protocolwhich has a completely different concept and role. Anthropic's MCP allows LLMs to use the accessIt's an interface for LLMs to access external tools or data. It's a one-time call-based input extension tooland is model-driven in design.
Outcode's MCP, on the other hand, empowers agents to divide roles, collaborate, and execute execute workflowsexecute workflows operations-centric architecturewhere agents share roles, collaborate, and execute workflows. It includes message passing between agents, conditional branching, exception handling, and even human collaboration.
In other words, Outcode can write to external MCPs, but it is not a complete Agentic Execution Platformin its own right.
โ
โ
Why Enterprise Agentic Platform?
For organizations to truly benefit from AI, they need to have the following elements in one unified structure
- Analytics and predictive capabilities (Data + ML)
- Situational awareness and judgment (LLM + Rule Engine)
- System integration for execution (iPaaS-based integrations)
- Human-in-the-loop collaboration structure
ALL OF THIS MUST BE NATURALLY CONNECTED AND OPERATIONALIZED SO THAT AI CAN BECOME AN OPERATIONAL ENTITY THAT ACTUALLY DOES THE WORK OF THE ENTERPRISE.
โ
Outcode provides a true Enterprise Agentic Platform that fulfills all of these requirements.
The foundation we are talking about is not just the ChatGPT API.
It is a structured AI platform optimized for enterprise operations, with Agentic Workflow, Multi-Agent Collaboration, and iPaaS-level integration infrastructure.
Outcode is at the center of this transition, helping companies realize AI-driven operational transformation.
โ
Business automation for enterprise operations
Get an introduction to Outcode, enterprise use cases, and expert advice all in one place